Table of Contents
Navigating the data vendor landscape, Explorium ensures high-quality data discovery and integration through a meticulous process. This involves market analysis, rigorous data validation, and compliance with legal and security standards. By evaluating sources for coverage, accuracy, and freshness, Explorium guarantees that onboarded data meets the highest standards. Here, we answer common FAQs about Explorium’s comprehensive approach to delivering top-notch data solutions.
Research
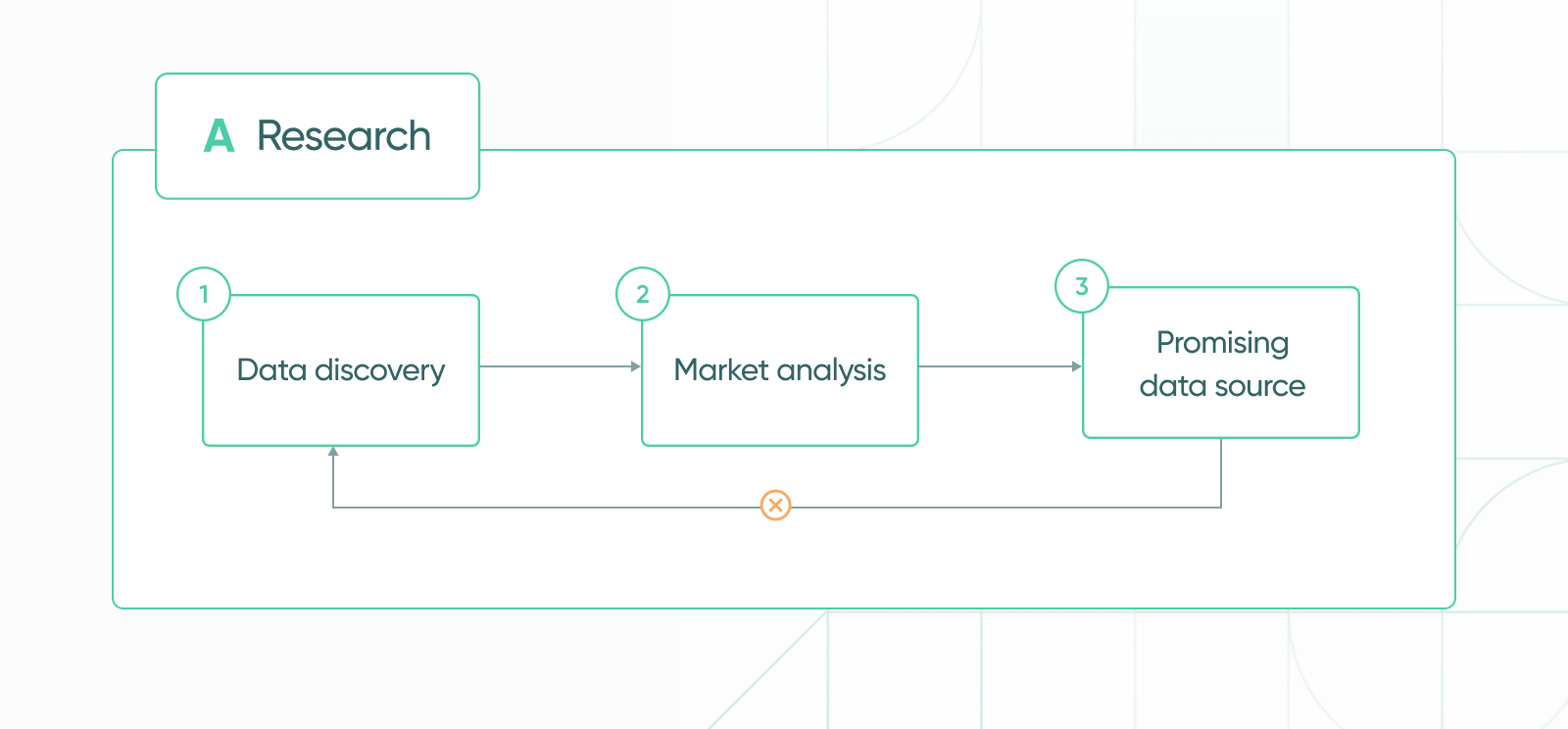
(1) Data discovery: mapping the ever-changing and evolving data vendor landscape. Detecting the initial leads and offerings that answer strategic and tactical needs of Explorium’s current and potential customers.
(2) Market analysis: Explorium initially compares several potential partners and sources providing similar data on aspects such as coverage, accuracy, freshness, and uniqueness to gain the best perspective of what data is available on the market. Although two sources may sell the same type of data, their coverage and area of specialty may differ. Comparing where two sources overlap and compliment each other allows for a holistic decision regarding which sources will be thoroughly evaluated and possibly acquired. E.g. a source may provide contact data for SMBs, and another for fortune 500 companies.
(3) Data sources consist of either third-party data partners or open-source data. Sources that have the potential to provide unique data are thoroughly evaluated.Our partners are market leaders in the data vendor sector. Explorium examines many vendors based on recommendations and requests from design partners and customers, in addition to data hunting and exploration efforts.
Evaluation
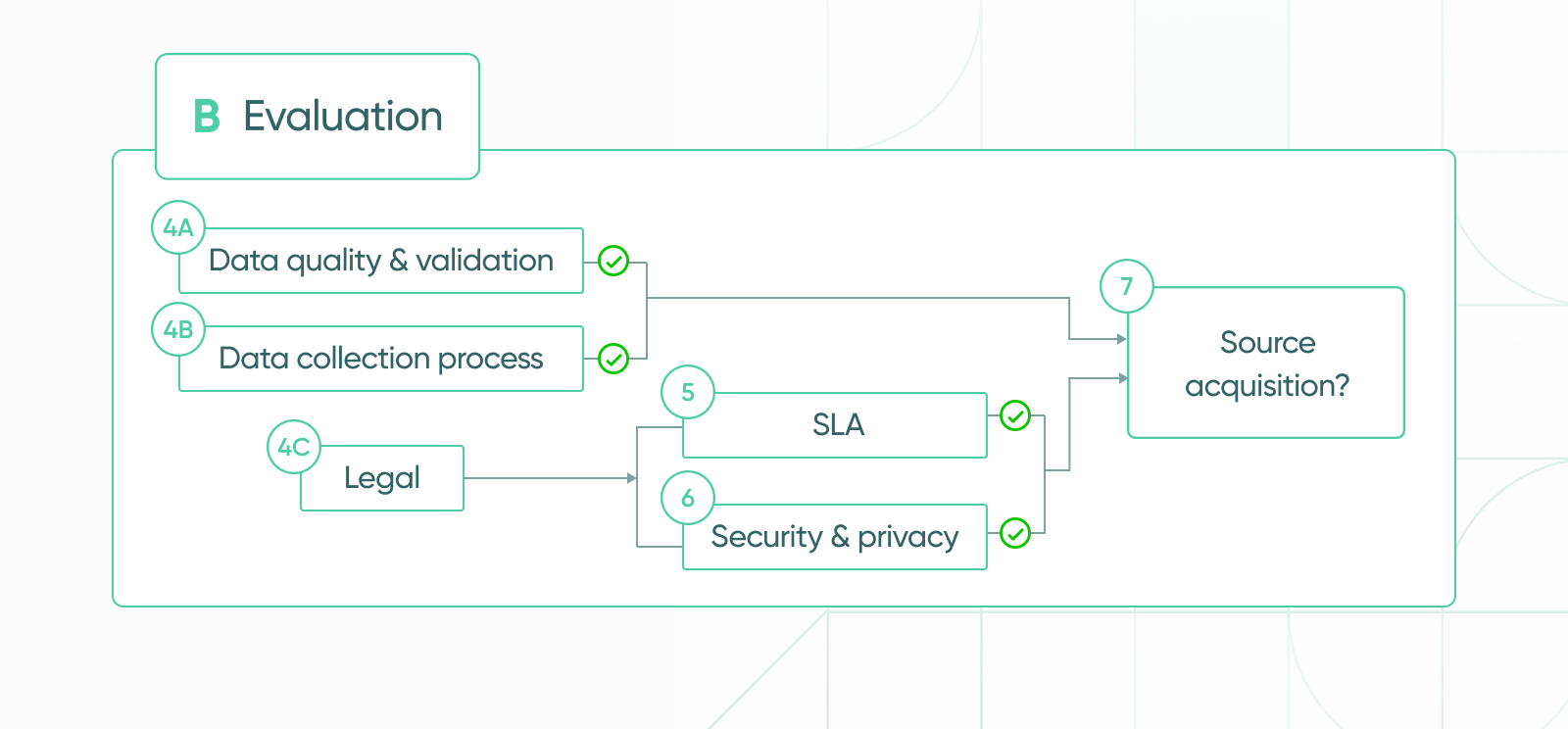
(4A) Data quality & validation: analysis of the sample data provided by the potential partner. Sample data is cross-checked with ground truth existing sources that are curated through a variety of methodologies. The analysis of the sample data provides an overall understanding of how the partner balances the tradeoff between accuracy and coverage.
(4B) Data collection process: understanding the potential source’s data collection methods is critical in examining the potential source’s quality. E.g. modeled data, survey data, and aggregated data are tested differently.
(4C) Legal: in the legal stage, if the source is a potential data partner, commitments to security, privacy, and SLA are finalized. These aspects are examined before entering the legal phase, however Explorium requires a legal commitment to continuously assure proper treatment of security, privacy, and SLA.
(5) SLA: assuring longterm availability of attributes and signals, support provided from partners, format negotiation, optimal latency and QPS (queries per second) conditions.
(6) Security & Privacy: assuring partners comply with the
relevant audits, reports, laws, regulations (e.g. GDPR, CCPA) and standards (e.g. ISO-27001, SOC 2 Type 2) per regions, countries, and data type. Explorium has SOC2 Type 2 audit reports, and complies with the following certifications: ISO-27001 Information security, ISO-27701 Privacy, and ISO-9001 Quality. For additional information visit https://explorium.ai/data-security/.
(7) Source acquisition decision point: onboarding open-source data or acquiring third-party data from a newly minted data partner that passed all evaluation tests.
Onboarding
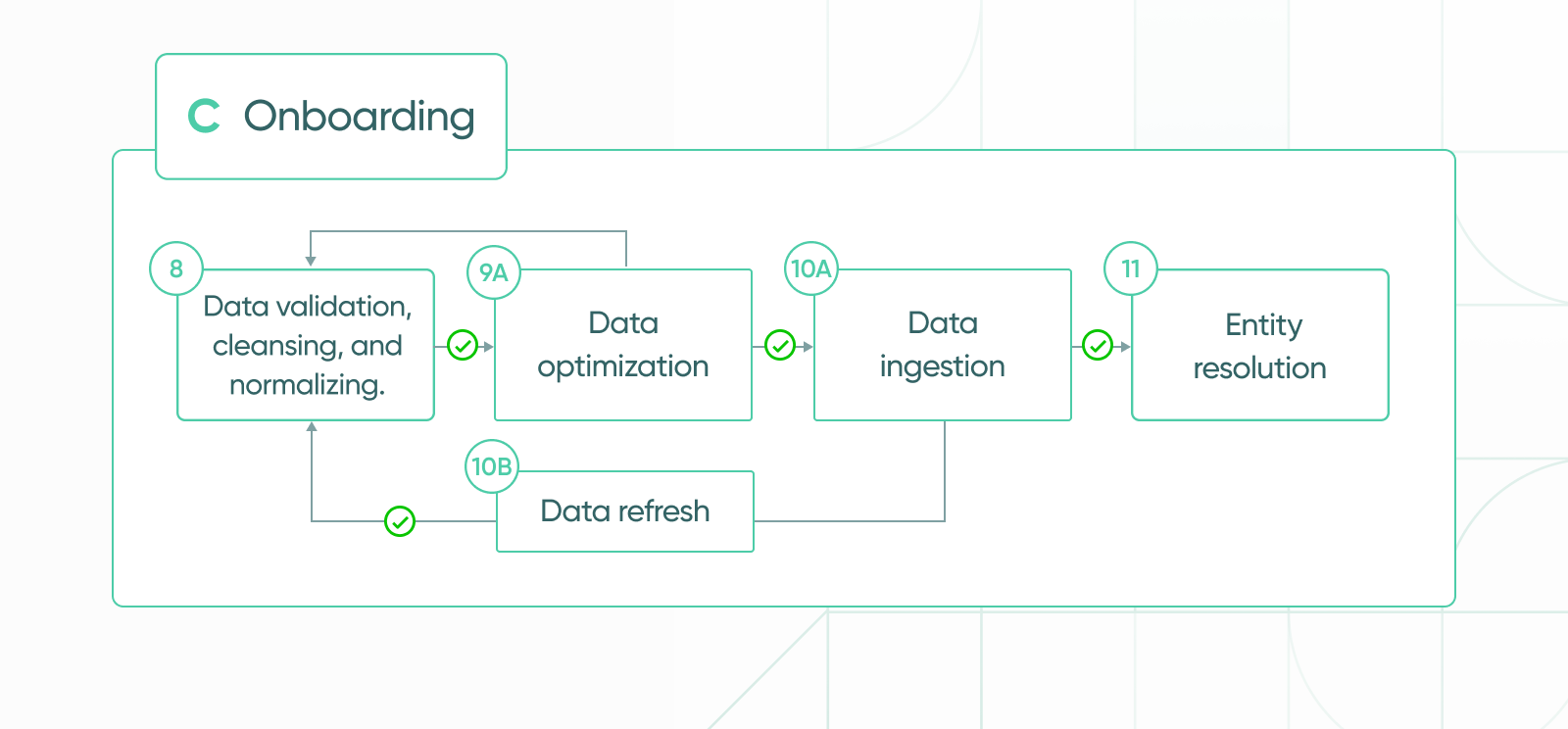
(8) Data validation, cleansing, and normalization: validating the onboarded data has the same quality and accuracy of the original sample data that was analyzed pre-onboarding. Cleansing the data of incomplete or incorrect records, a process that includes detecting the errors and then replacing, modifying, or deleting the corrupted records. Normalizations improve the integrity of the data, allowing the developers to structure the data in a way that aligns with Explorium’s matching logic, in anticipation of the onboarded data pairing with customer data.
(9A) Data optimization: understanding the highest impact value of the data supplied by the source. Using a variety of methods such as feature engineering to restructure and improve the data to extract the most relevant derivatives for ML projects.
(9B) Validating optimized data: monitoring that the optimized data is valid.
(10A) Data ingestion: uploading and processing the new data into Explorium’s data lake. Data lake repositories are where data is stored and secured.
(10B) Data refresh: onboarded fresh data from the source is revalidated, re-cleansed, renormalized, and re-optimized. Refresh rates depend on factors such as the data’s use-cases, sources, quality, and more. Many of Explorium’s sources are refreshed quarterly or live-on-demand.
(11) Entity resolution: running entity resolution algorithms to pair identical entities across Explorium’s sources. Enriching the onboarded source with new entities to increase Explorium’s coverage and accuracy.
Development
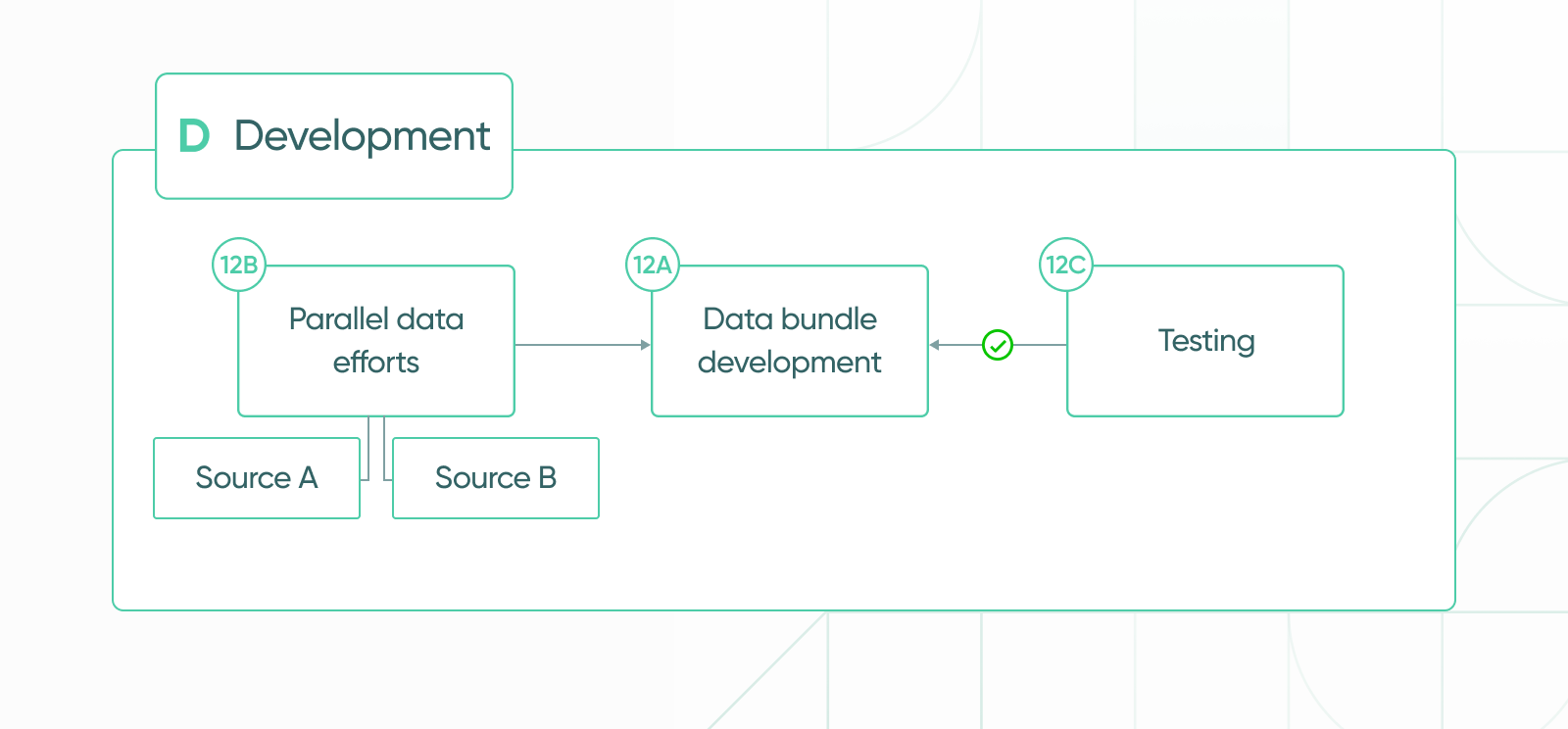
(12A) Data product development: a typical data product includes an amalgamation of data acquired, onboarded, and combined from a variety of sources. Explorium develops proprietary data that is created via aggregations, optimizations, comparisons, modeling, GenAI, and much more to best serve our customers’ and design partners’ use cases.
(12B) Parallel data efforts: each source that is combined to create a production-ready data bundle must be evaluated, acquired, and onboarded separately.
(12C) Testing: during the development phase the new data bundle undergoes testing and Q&A. This stage includes pre-release testing involving trial tests of the data both internally and by Explorium’s design partners.
Delivery & Monitoring
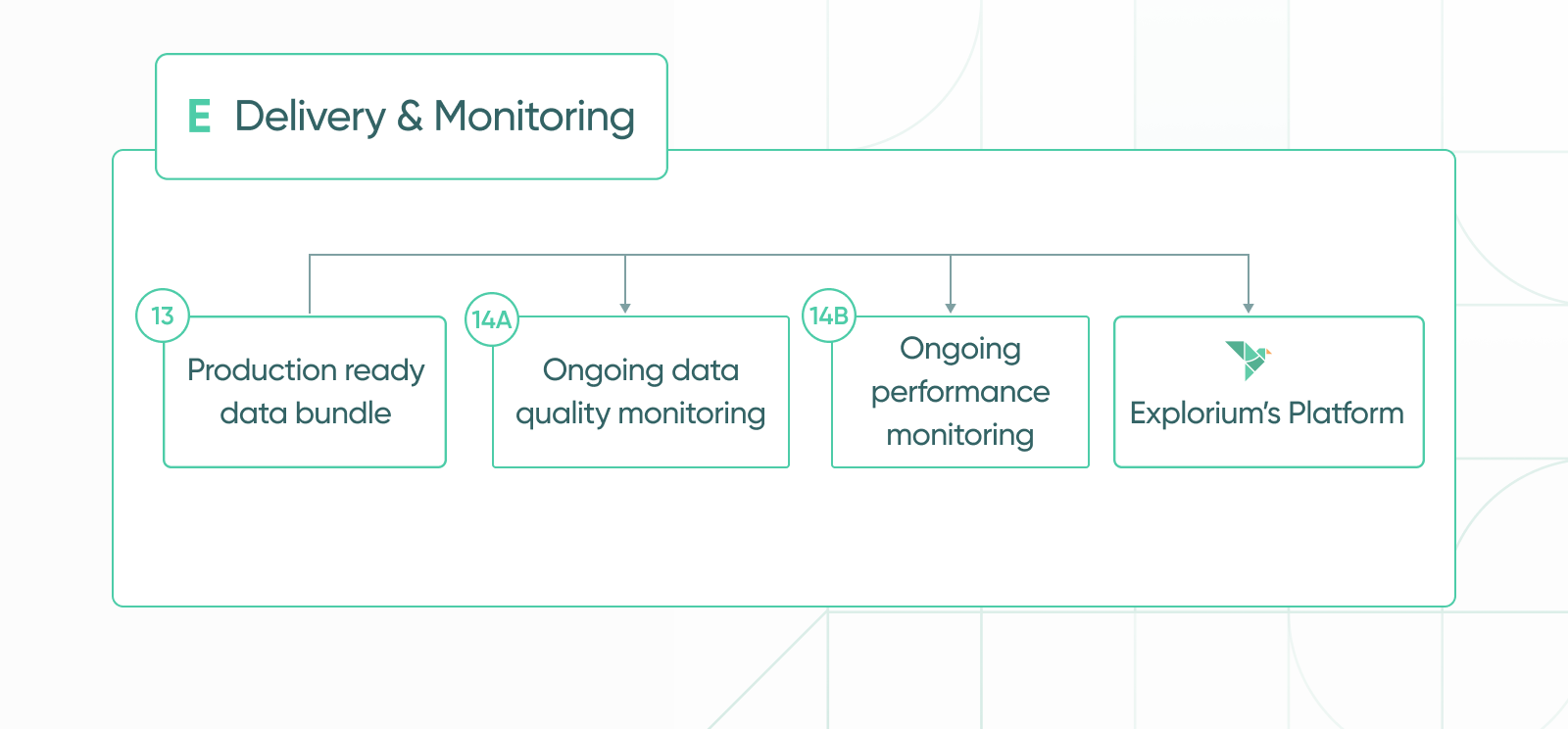
(13) Production ready data bundle: data is on platform and ready to be consumed by customers!
(14A) Ongoing data quality monitoring: assuring Explorium’s production-ready data meets data quality benchmarks, such as coverage, accuracy, and more. Quality assurance incorporates a combination of manual, automated, machine and AI methodologies.
(14B) Ongoing data performance monitoring: ongoing maintenance assuring the data is performing according to the contractually agreed upon SLA including data latency and load. Continuously developing improvements for data delivery mechanisms.