Table of Contents
Introduction
Our economy has experienced many periods of growth and decline affecting the Manufacturing sector. The outbreak of COVID-19 has had a negative effect on this sector with revenue declining in 2020, driven by lockdowns and supply chain disruptions. While various factors as well as government financial assistance helped manufacturers recover and even surpass the pre-pandemic level by the end of 2021, in the US, the sector’s revenue has been declining at a CAGR of 0.4% to $7.0 trillion over the past five years, including an estimated 2.0% decline in 2023 alone. In 2023, industry profit is projected to decline to 8.0%, a staggering drop from previous years.
These ongoing challenges for manufacturers have been exacerbated by inflation-driven, rapidly rising prices affecting everything from distribution costs, and business closures. This climate makes it more crucial for manufacturers to penetrate new markets and sectors they may have previously neglected, and to prioritize the right targets in those sectors.
The questions this white paper addresses are how can manufacturers accurately identify their TAM – total addressable market – that is, identify all the potentially relevant B2B customers and intelligently prioritize them. It presents Explorium’s Predictive Prospecting solution, which is part access to vast, diverse, comprehensive data and part machine learning capabilities used to build powerful, predictive lead scoring models. Explorium’s solution is used by manufacturers to drive efficient growth through their field sales organization.
Key growth challenges facing manufacturers
In a research study conducted by the Manufacturers Alliance, hundreds of executives listed competition, market conditions, and pricing as key challenges facing field sales today.
When asked how they would solve these challenges, a majority named cost reductions and sales process improvements. However, many were quick to mention that cost reductions were out of their control, but focusing on sales process improvements was their ticket to more successful outcomes.
Top solutions to industry challenges
At Explorium, we focus on just that – helping manufacturing executives expand their traditional markets and enter new ones too – by ensuring that their sales teams have the information they need to discover and reach out to high-quality prospects that will drive real growth and revenue, and move the business beyond the competition.
Predictive Prospecting for Growth
Populating your TAM with all the relevant businesses
The cornerstone of successful sales efforts is the accurate mapping of all the potential customers (leads) in a designated territory, followed by the ranking of leads in the territory according to criteria relevant to the manufacturer. When salespeople have incomplete or incorrect information about potential customers in a specific territory, they waste their time on irrelevant leads and miss out on real and good opportunities.
One part of the challenge in identifying all the relevant businesses in your TAM – total addressable market – is the changing landscape of opening, closing, and moving businesses, particularly in the current inflationary climate. Complicating the task even further is scarce data about SMBs – small and medium-sized businesses – which comprise more than 90% of all businesses, and are super relevant to manufacturers. For example, categorizing a type of business should be straightforward. Is it a warehouse or a small-scale tool manufacturer? This is often where the size of the business comes into play. Accurate information about SMBs is hard to come by.
In general, they are not legally required to publish reports of their activities or revenue; they may not have websites and perhaps only show up on Facebook or LinkedIn, or nowhere at all.
This information gap is why it is so hard to locate, for instance, all the metal-cutting workshops in a territory, a specialty that includes many small businesses. It very frequently leads to them not being classified correctly or consistently in industry classification sources like the NAICS code.
Thus, the lion’s share of the information problem stems from insufficient data points. There are many more factors beyond, for instance, company size and revenue, that are required to identify businesses in your TAM. Many companies typically use only very few, limited, and elementary types of criteria to properly characterize their addressable market. This means that other and varied sources of information must be accessed in order to discover information about businesses that could be good prospects. Back to the example above, to properly classify the industry to which a business belongs, a good predictive prospecting solution would cross-reference and validate the industry classification by using the NAICS code but also add the business category, segment, and other sources, to zero in on relevant leads.
Populating your TAM with all the relevant businesses
Without the right data, sales teams are unaware of all the businesses in their territory that exist and that are relevant prospects. The view of the territory is foggy.
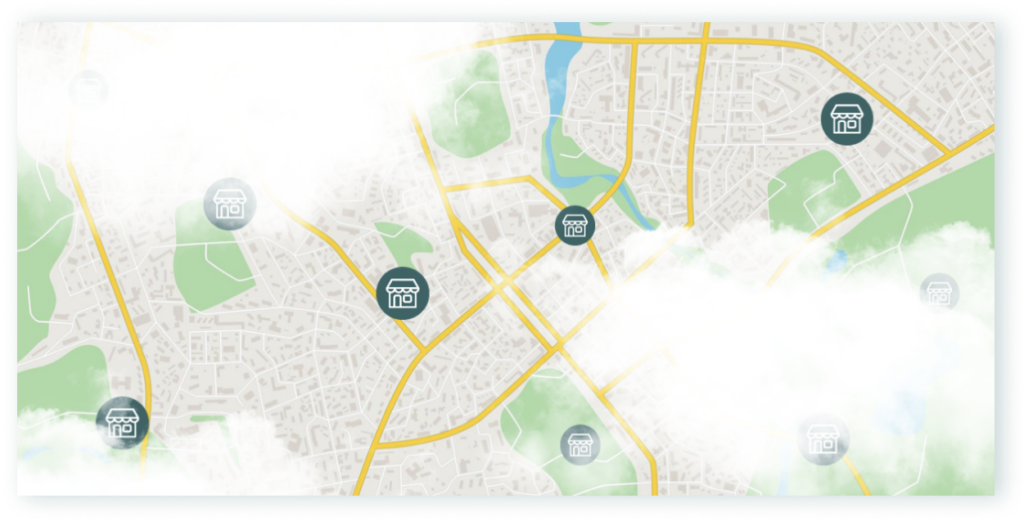
To capture not only a real picture of your TAM but also of the relevant customers within it (i.e., your ICP- ideal customer profile),you need a solution that provides access to the data and the tools that enable you to go beyond the elementary attributes of a company and supplement your existing data with new signals like employee YoY growth, departmental growth, technographics, website visitors, social media profiles, customers reviews, number of locations, and (thousands!) more. This kind of data helps you hone in on more businesses that match up with the type of customer you are seeking.
More specifically, getting back to identifying metal cutting workshops relevant to your business, looking at specific keywords on their website or other public-facing sources, the machinery used (e.g., TrueBlend Metal Cutting Machine, Granulator X Series Metal Cutting Machine, Rapid 600 Metal Cutting Machine), employee skill-sets (e.g., CNC milling or turning operators) or specific products they provide (e.g., jet engine parts, knee replacement implants, or wind turbines) can all be excellent indicators that this is a relevant prospect for your business. They go above and beyond company size and revenue, providing highly informative data.
Prioritizing your prospects and focusing on the right ones
Because many businesses could potentially be good leads, prospects must be prioritized. This is crucial so that salespeople do not waste time, and instead approach only the leads that are more likely to convert to customers first.
The likelihood to convert is just one attribute you may want to predict about potential customers. What about their LTV – lifetime value – over the next few years, or their likelihood of churn?
Identify the right prospects for the long term
Can you see a clear picture of your market and predict unique attributes such as likelihood to convert and LTV about your potential customers?
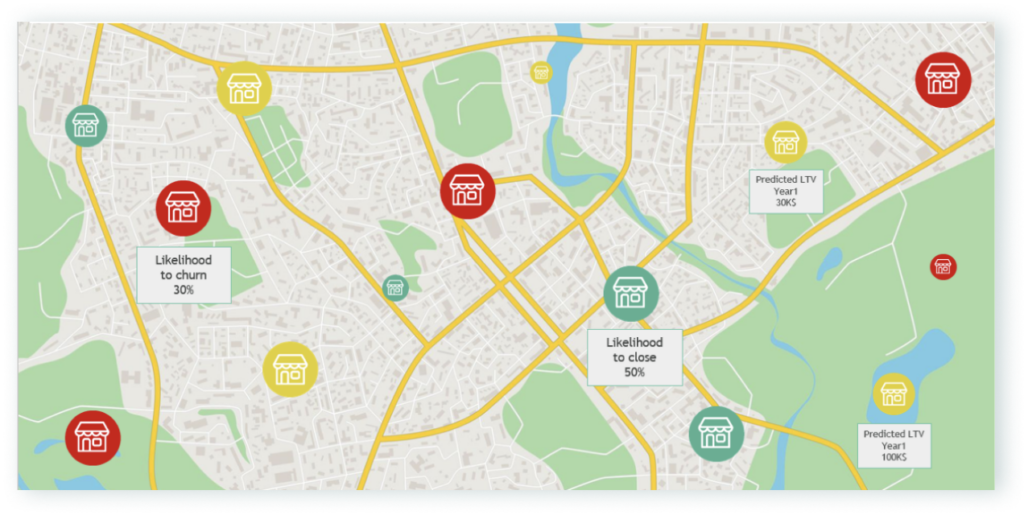
When we talk about prioritizing leads, we are referring to a machine learning process which uses mostly external data to score leads.
Scoring your leads to prioritize them is a data science challenge. Even if you, the manufacturer, possessed all the relevant, distinctive data points relevant to your leads, you would need a data science team to process the data for sales and marketing efforts. You probably have data science experts on your team that deal with production, but not necessarily with company growth.
Dedicated data teams are a necessity for dealing with new external and existing data you gather about prospects. External data needs to be matched and integrated into your CRM system. On top of that, a sophisticated scoring model must be built which meets your unique business criteria. Most companies settle for rules-based scoring models, but this is not enough.
To go beyond basic models and make sure your scoring model provides the competitive edge you want, machine learning capabilities are needed that allow you to continuously analyze your win/loss data and discover the attributes that define a quality lead. Such leads are the cream of the crop and have a higher probability of being a good match for your products and services and hence, are more likely to convert
Conducting the search for accurate external data
he route to identifying all the relevant businesses in your TAM and prioritizing them relies on data – mostly external data. Procuring and processing external data is a complex challenge.
Certainly, marketing, sales, and business development teams have a very good idea of their market, and the types of businesses they have and would like to have in their customer base.
In seeking out external data with signals they believe will be valuable, they usually have a checklist and search for the providers with the applicable datasets, and eventually contract with them. Post-purchase, the new external data must be integrated with the team’s own internal data and system.
Though the steps to external data acquisition may be clear, the process of acquiring even one dataset takes several months. There is a profusion of choices offered by many different vendors, and each dataset must be manually evaluated. Furthermore, there are key issues that must be clarified in advance, including ensuring the data complies with GDPR and other relevant regulations. In addition, since data becomes stale quickly, the vendor will need to ensure routinely refreshed data, as appropriate per the nature of the information.
The procurement process for each dataset acquired is also lengthy. Companies generally purchase a number of datasets since each one contains different attributes. Thus, it can be a costly process, particularly when companies work with an average of five external data sources.
Once the purchase has been made, it cannot be put immediately to work. The data must be matched and harmonized with the manufacturer’s internal data. Which may be used by more than one department. Data integration is complicated and time consuming and the data engineering team must take on this responsibility as a dedicated project.
After all the investment of time, funds, and work of three to six months per dataset, there is no guarantee that it will deliver the added value and impact sought. Unfortunately, failures do frequently occur, whether due to the dataset, the manner of integration, utilization, or other reasons.
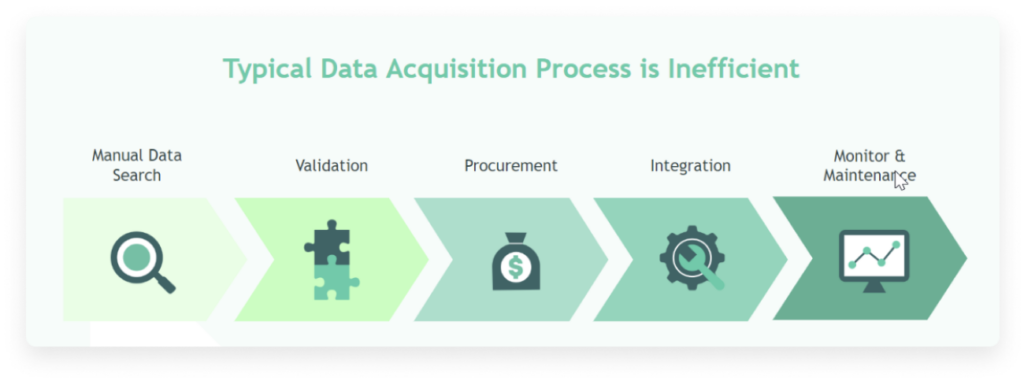
The lengthy data acquisition process requires data specialists to be involved. This can deter companies from moving forward on such projects.
Still, manufacturers and their sales teams understand the importance of expanding their reach to good prospects in existing and new markets and are looking for ways to embark on such endeavors, particularly in the current economic climate.
Explorium’s Predictive Prospecting Solution
Explorium’s Predictive Prospecting solution gives manufacturers an all-in-one means to grow their business through a platform with access to thousands of data signals about millions of businesses together with the algorithmic tools to develop machine learning models that predict which potential customers make the best prospects.
The How-Tos of the Explorium Solution
The process of locating all the possible customers in your market, then selecting the truly relevant ones, and prioritizing them is explained below as a multi-stage process – While multi step, this process occurs within mere minutes from the time a business name enters your system to when it lands in your CRM, loaded with information and a lead score.
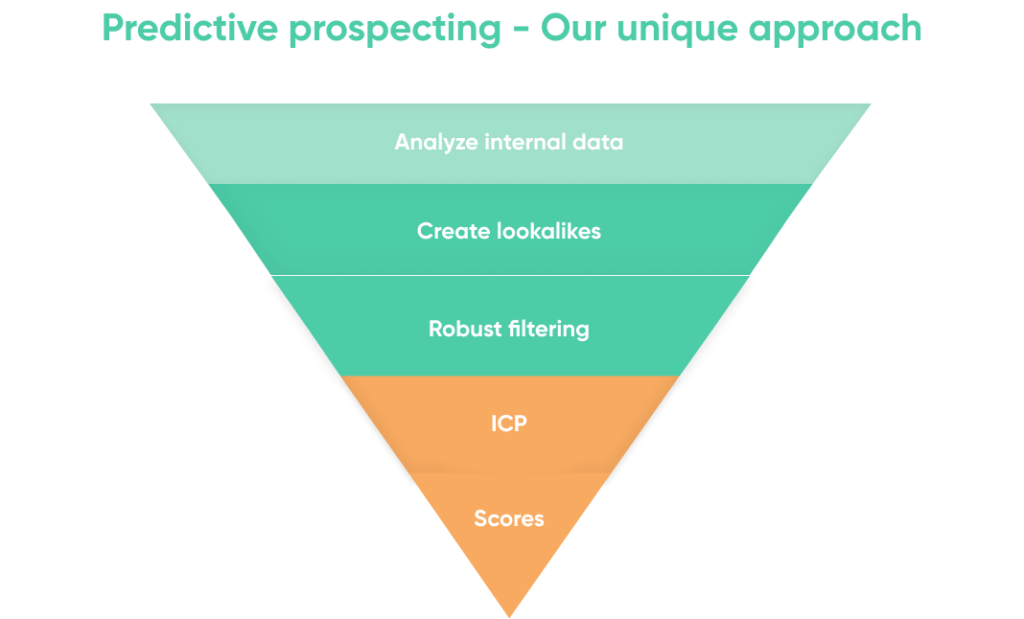
Predictive Prospecting framework used by manufacturers to generate dependable and scalable results.
Data-related actions
Analyzing internal data – valuable information gleaned from your internal systems.
The initial step examines the existing data in the company’s CRM, collecting existing customer attributes, and zeroing in on those that distinguish them from businesses that are not customers. A deeper analysis makes finer distinctions among existing customers conducting, in effect, a win-loss analysis. For example, in selling parts to a chemical plant, is there more success with polymer factories or pharmaceuticals? Which more easily converts, buys more, with greater frequency, etc.?
Create lookalikes. Imitation – the highway to new customers.
In the next step, many more businesses in the TAM are added to the system by creating a list of lookalikes, that is, businesses with attributes like those of existing customers. Using Explorium, companies gain immediate access to more than 4,000 data attributes covering roughly 135 million business entities worldwide. With such incredibly broad access to data about businesses – small, medium, and large business – the list of lookalikes you can create is wide and comprehensive and no relevant business in your TAM is left out. Our customers use our one-stop data platform to access a large selection of data bundles. This means no months-long wait to procure, examine and test data and no set-up issues.
Dedup – cleaning up the dataset.
Now that you’ve added many new lookalike businesses to your list, you will need to get rid of some duplicates. Our platform uses fuzzy matching and entity matching across both internal manufacturers and external Explorium-sourced datasets to identify and eliminate duplicate records for businesses. It is not unusual for businesses to have more than one entry in databases due to slight variations in address or corporation type. For example, is “Chemical Plant, Inc.” the same business as “Chemical Plant, Ltd.”? By analyzing a range of information and sources, the entity matching process answers that question.
Robust filtering – zero in on the prospects in your ICP.
With Explorium, manufacturers gain access to thousands of attributes about each potential customer. These attributes are used to zero in on the right prospects for you.
Industry categories
Industry categories as well as sub-categories are core pieces of data that determine whether businesses are included in or eliminated from the target list. In many cases the classification is wrong – for example SMBs are hard to categorize and might not be classified, or have an incorrect one – a business that uses robotic manufacturing may be categorized as a business that manufactures robots; emerging industries, for example those involved in renewable energy, may not yet be classified and/or may not be classified correctly in the traditional sources. The Explorium solution detects and confirms the industry classification using a number of sources; as a result, you get more coverage and can choose the classification sources that are best for your use case.
Website keywords
Using Explorium you can also conduct searches of target businesses’ websites looking for keywords deemed essential to discovering whether the target businesses possess the attributes you are seeking – for example, all factories in a specified area using specific industrial machinery.
The comprehensive and iterative filtering process pares the list down to the businesses with the highest conversion probabilities. The businesses included reflect the manufacturer’s ICP – ideal customer profile – or simply put, all the businesses with the features most similar to those of existing customers.
Manufacturing Case Study
How Explorium Helped a Manufacturing Giant to Uncover Millions of Dollars in Net-new Opportunities –
Background
Our customer, a multinational industrial manufacturing company with operations worldwide, engaged Explorium to help them execute a growth plan that required identifying new territories and high value prospects within a saturated American market.
Three challenges facing the customer
At the end of the process, the validated and thoroughly analyzed data is ready to be fed into the scoring models using Explorium’s machine learning engine. The resulting lead scores intelligently shape future sales efforts in the relevant TAM.
- The company needed a reliable way to identify and map all high potential customers. Their goal was to create a detailed list of prospects in the Midwest according to their specialized metal work criteria.
- They needed to locate reliable data in a quick and scalable way. Fast access to industry-specific data was a make-or-break for them.
- With a large sales force, it was key for their salespeople to use their time effectively and to have complete and correct information about prospects.
Predictive prospecting: High intent leads result in high ROI customers
Explorium’s predictive prospecting solution focuses companies on high intent leads that convert to longer term and high ROI customers.
For this manufacturing customer, 10,000 high value leads were identified. The projected value of converting those leads is tens of millions of dollars in the pipeline.
Breaking down Explorium’s process
Data-related actions
Using Explorium, our customers gain immediate access to roughly 135 million business entities, and more than 4,000 unique data attributes about them.
This manufacturing customer focused on:
Machine learning-related steps
They were able to score, prioritize, and identify their high-value prospects using our robust analytical capabilities.
Summary
Honing in on the right potential customers in your TAM and prioritizing them is a perpetual growth challenge in field sales. With Explorium’s comprehensive external data and ML-driven solution, though, the route to reaching the businesses you seek has never been smoother and less resource-intensive.
ML-fueled models built on the Explorium platform give manufacturers the tools to turn raw data into valuable insights that help them identify all relevant businesses in the TAM and prioritize them with fact-based lead scores. Your sales teams can confidently pursue the leads most interested in your product categories.
Explorium’s data, methodology, and strong predictive prospecting capabilities help manufacturing executives beat their competition by working to ensure that their sales teams have access to high-quality prospects that drive real revenue.